This section lists my curriculum development activities in information science and data science,
as well as individual course development & teaching work, complemented with a selection of associated student evaluation reports.
- Designed: Specialisation Data Science (2022): Population Health Management (LUMC)
-
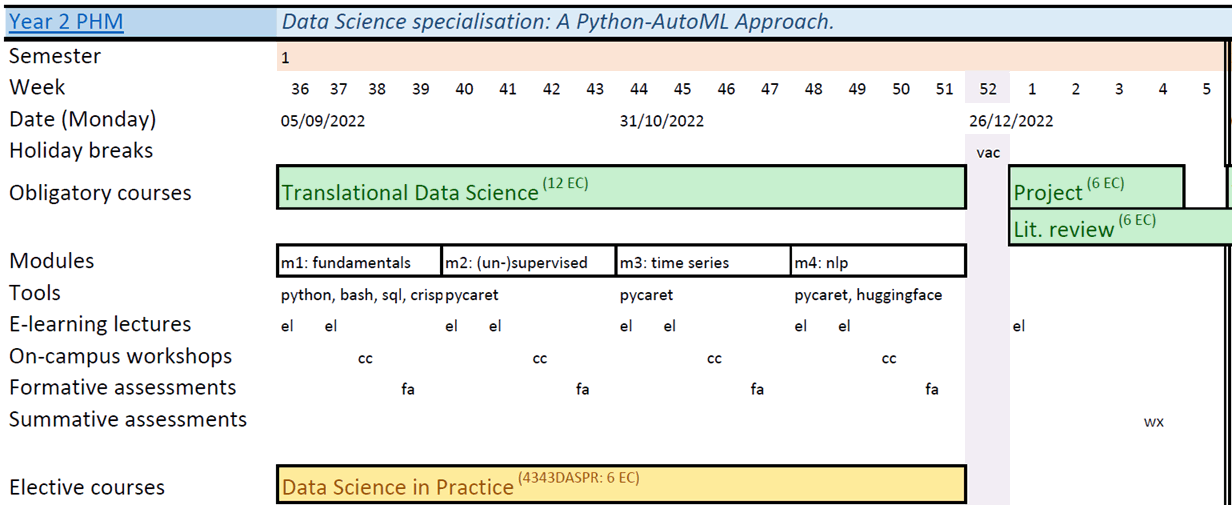
The TDS Lab designed a second year specialisation track in the Population Health Management (PHM) MSc curriculum, by taking a novel low-code approach to maximally and reliably facilitate employing state-of-the-art data science solutions for students without a computer science background. However, this track could not be implemented.
- Co-designed: Master's profile ADS (2016-2020): Applied Data Science (UU)
-
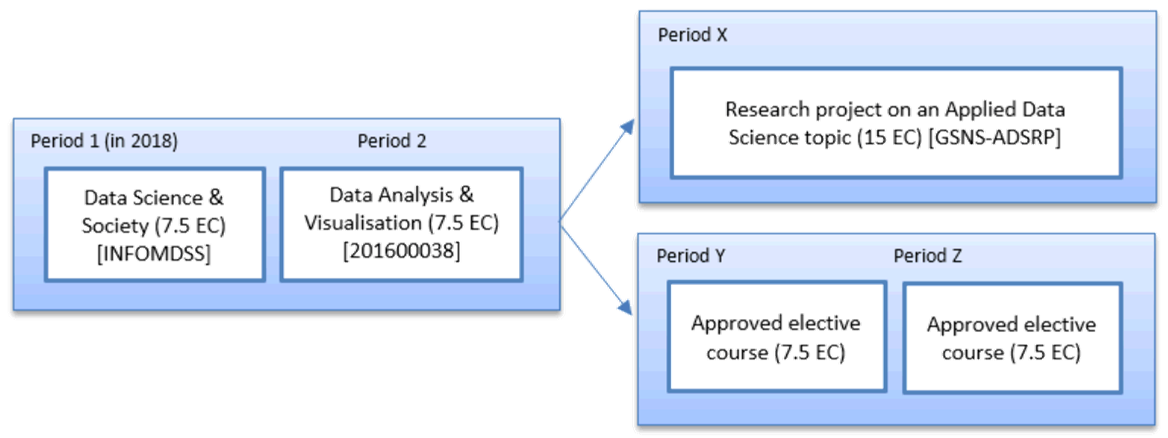
I have co-created and coordinated the master's profile Applied Data Science at UU's Graduate School of Natural Sciences (GSNS) and Graduate School of Life Sciences (GSLS). Together with a UU-wide ADS working group we defined the learning objectives and the curriculum content of 30 ECTS for students from GSNS and GSLS backgrounds. The ADS master's profile comprises two mandatory multidisciplinary courses (15 EC) complemented with either a research project (15 EC) OR a selection of 2 elective courses (15 EC) from the elective courses table listed under B2. The illustration below visualises the Master's profile Applied Data Science.
I concluded the specification process by writing the official Education and Exam Regulations (OER) annex 2016-2017 (Applied Data Science Profile, pp.69-71).
- Co-designed: Internal certification PADS (2016-2020): Master Applied Data Science Postgraduate (UMCU)
-
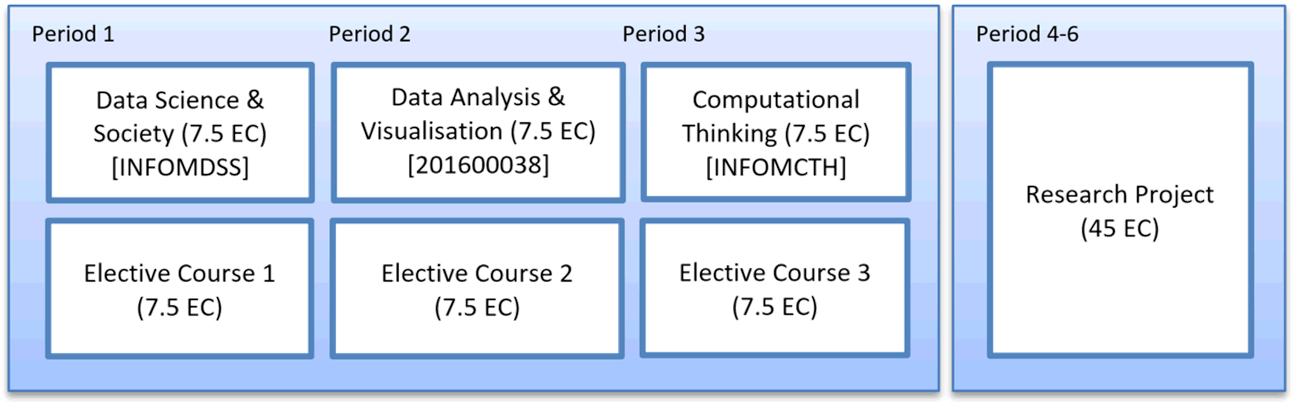
I have coauthored the UU-internal certification proposal for a 90 ECTS, post-graduate MSc programme Applied Data Science for Health, with the Health Sciences CROHO label 75054, hosted by GSLS, which subsequently launched in September 2017. Its unique selling points are fourfold. First, this is the first postgraduate MSc in the Netherlands that does not focus on business analytics, but on the application of data science in the field of health. Second, its multidisciplinary character connects Statistics and Informatics perspectives onto Applied Data Science on par. Third, it's embedded in the new UU focus area Applied Data Science and its active data science community. Fourth, it directly leverages top research in UU Faculties of Science, Social and Behavioral Sciences, and Medicine.
I have been both ADS master's profile coordinator and ADS MSc postgraduate programme coordinator from the start of these programmes.
- Designed: CUrriculum REvision: CURE (2013-2015): Bachelor Information Science (UU)
-
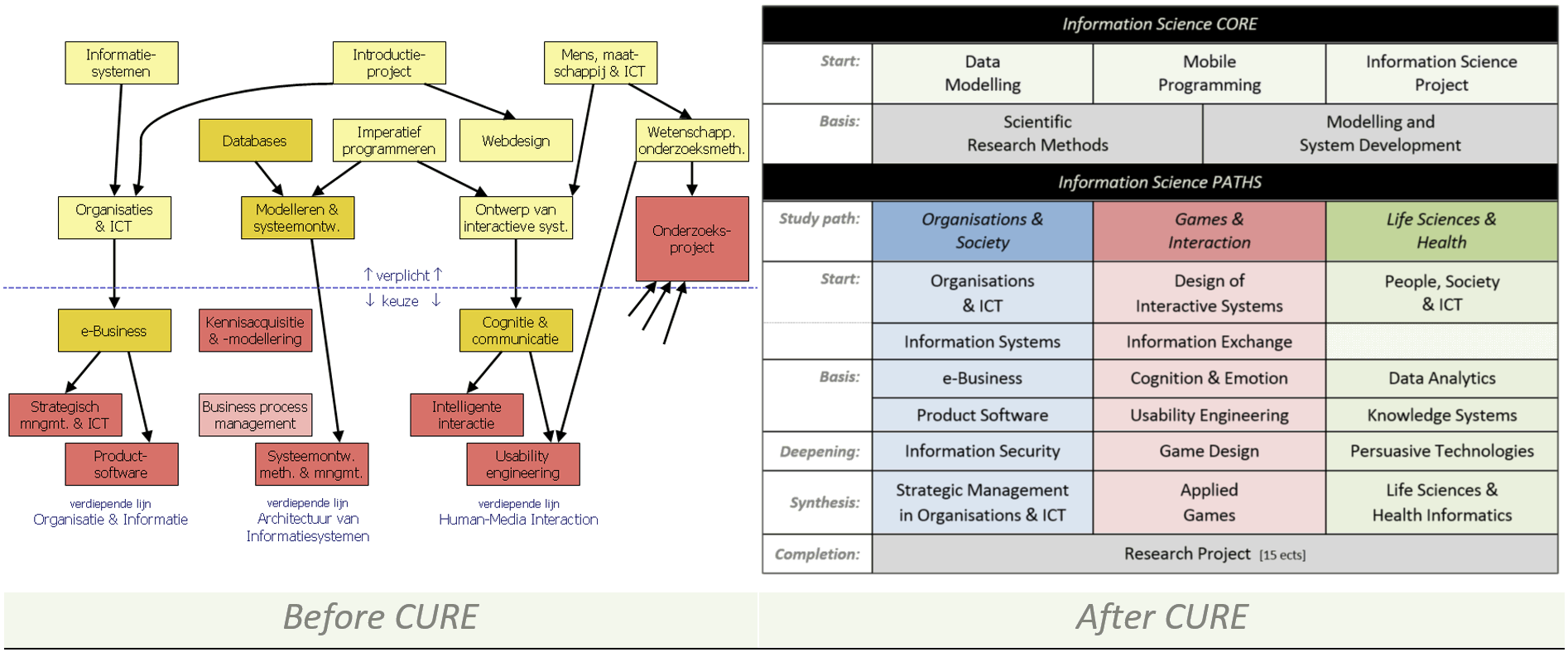
I have developed and supervised the major CUrriculum REvision (CURE) of the Information Science bachelor programme at Utrecht University. Its fourfold aims were to improve its ranking in the yearly NSE student evaluations, to follow up on the accreditation committee's recommendations, to better accommodate the many recent staff changes, and to strategically align the programme with faculty, university, national and European research agendas. At its core are three newly defined study paths which closely match the four secondary Dutch school profiles by design.
Existing courses were either recategorised, repartitioned, upgraded or removed, and new courses are being developed to help implement the new strategic focus, and to better connect the curriculum with current research. This was partly carried out in four staff-based workgroups.
I concluded the revision process by rewriting the official Education and Exam Regulations (OER) text (BIJLAGE D: Informatiekunde, pp.36-40).
- Designed: Data Science & Society (2016-2020): MSc level | 180 students
-
This is the introductory and mandatory course in all emerging Applied Data Science programmes. Its objectives are for students (1) to understand the role of data science and its societal impact, (2) to recognise the knowledge discovery processes in applied data science, (3) to identify trends and developments in big data technologies, (4) to apply big data technologies such as Hadoop to solve real-world problems, (5) to analyse unstructured data using natural language processing techniques, and (6) to understand the need for self-service data science.
Highlighted elements: A popular Data Science book review and pitch event; A data science platform market research; a mid-term and end-term assignment with a big dataset to solve a real-world problem for domain experts, currently employing the technologies such as Hadoop MapReduce, Apache Spark, NLP and AutoML. The conceptual Venn-diagramme relates the key topics in this course.
- Co-designed: Data Analytics (2015-2020): BSc level | 140 students
- Highlighted elements: Two Data Analytics project competitions in 3-person teams to actively learn the complete CRoss-Industry Standard Process for Data Mining (CRISP-DM) as applied in the Life Sciences & Health domain, from brainstorming sessions to conclusive project pitching event and a written CRISP-DM project report, respectively. For the 2017 edition we have notably been awarded a Teaching Award "in recognition of distinguished teaching".
- Designed: Business Intelligence (2012-2020): MSc level | 50+ students
- Highlighted elements: Running BI team project with working prototype, oral presentation and written report, scientific paper analysis talks, peer grading with statistical penalty system, participation grading, crash course DWH, regular mini-multiple choice exams which are taken, discussed and graded within the same workshop, guest lecturers.
- Designed: Life Sciences & Health Informatics (2014): BSc level 3 | 10 students
- Highlighted elements: Extra-departmental (entry-level) course in the broad domain of Life Sciences & Health Informatics as a course registration requirement.
- Designed: ICT in Life Sciences Innovation (2011-2012): MSc level | 30 students
- Highlighted elements: Required MOOC participation, brainstorming, feasibility analysis, market analysis, grant proposal writing, project pitching before external jury.
- Revised: Knowledge management (2009): MSc level | 35-50 students
- Added elements: Knowledge discovery & Knowledge infrastructure lectures.
- Revised: Method Engineering (2009): MSc level | 35-50 students
- Added elements: Linguistic Engineering workshops.
- Revised: Informatiesystemen (2009): BSc level 1 | 70-90 students
- Added elements: Four lectures, on Business Intelligence, Information Security, etc.
- Designed: Taalvariatie in Nederland (2006): BSc level 1 | 15 students (VU)
- Data Science & Society (UU, 2020-2021) [my score: 4.5/5]
- Master Business Informatics & Applied Data Science MSc Profile. Caracal INFOMDSS evaluation, by 45/125 students. Data science and society_Caracal_20689.pdf
- Data Analytics (UU, 2017-2018) [my score: 3.6/5]
- Information science. Caracal INFOMBIN evaluation, by 37/135 students.
- Data Analytics (UU, 2016-2017) [my score: 3.7/5]
- Information science. Caracal INFOMBIN evaluation, by 21/99 students.
- Business Intelligence (UU, 2015-2016)
- Business Informatics. Caracal INFOMBIN evaluation, by 15/52 students.
- Business Intelligence (UU, 2014-2015) [my score: 4.1/5]
- Business Informatics. Caracal INFOMBIN evaluation, by 18/32 students.
- Informatiesystemen (UU, 2011-2012) [my score: 3.81/5]
- Information science. End-term paper evaluation, by 67 students.
- Informatiesystemen (UU, 2011-2012) [my score: 3.86/5]
- Information science. End-term online evaluation, by 22 students.
- Informatiesystemen (UU, 2011-2012) [my score: 4.21/5]
- Information science. Mid-term paper evaluation, by 46 students.
- Knowledge management (UU, 2010) [my score: 4.13/5]
- Business Informatics. Generic course evaluation, by students.
- Method Engineering (UU, 2009) [my score: 4.0/5]
- Business Informatics. Generic course evaluation, by students.
- ICT in Organisatie, Beleid en Management (UU, 2008-2009) [score: 4.1/5]
- Information science. Generic course evaluation, by 38 students.
- Master thesis supervision (UU, 2007-2008) [score: 8/10]
- Business Informatics. Student evaluation, by students.